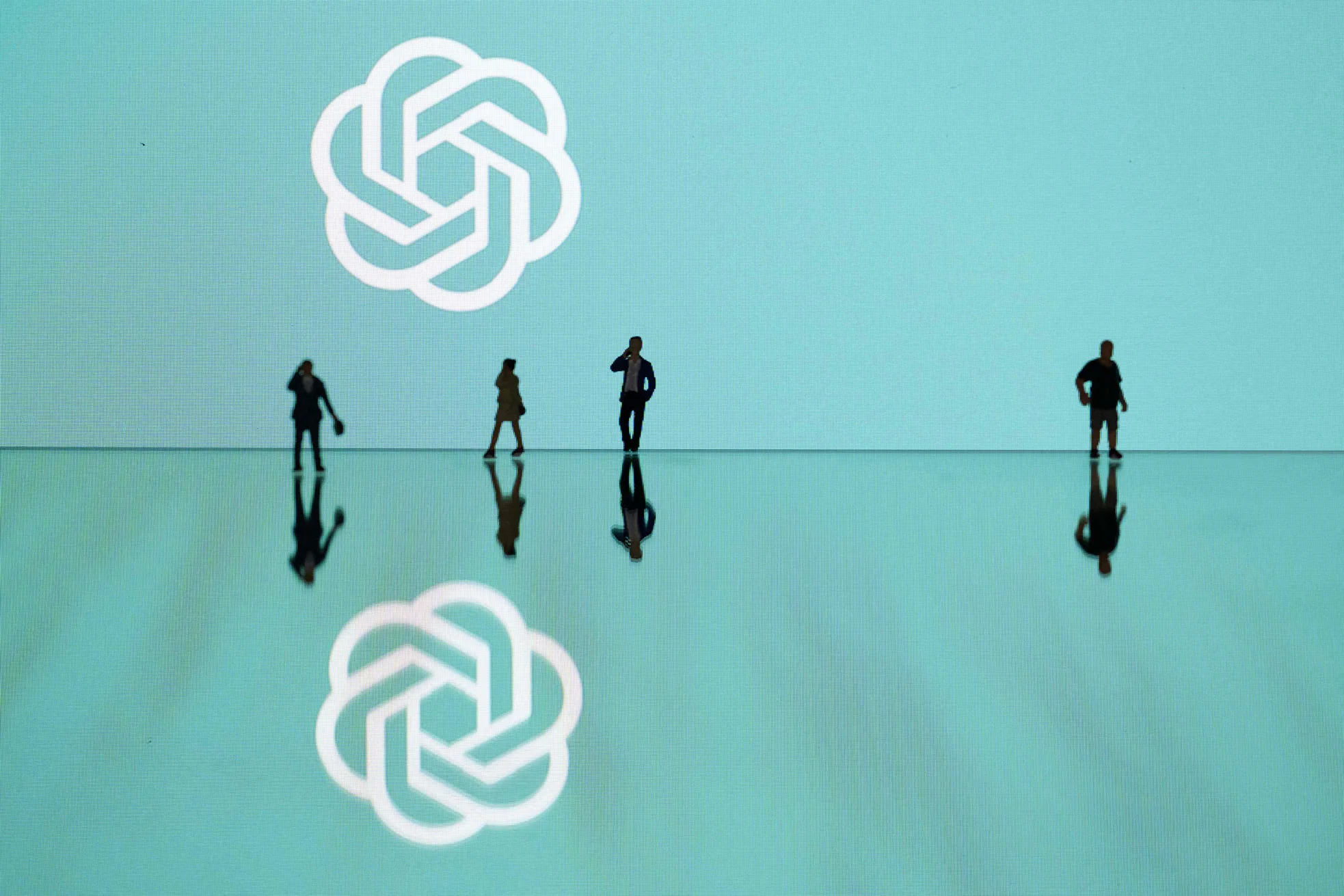
ChatGPT Sends Millions to Verified Election News, Blocks 250,000 Deepfake Attempts
AI
Zaker Adham
09 November 2024
07 August 2024
|
Zaker Adham
Summary
Summary
A team at the University of Toronto has developed an AI model that can understand how humans perceive creativity in chess. In a recent paper presented at an international conference, researchers from U of T's Faculty of Applied Science & Engineering detailed their use of game trees and deep neural networks to help chess engines recognize brilliant moves.
This innovation could lead to chess engines that prioritize creative and clever strategies over simply maximizing win rates, potentially influencing other AI systems involved in creative tasks.
"A chess move is seen as brilliant or creative when its strategic benefit isn't immediately obvious, but in hindsight, the player had to navigate a precise path through numerous possibilities," explains Michael Guerzhoy, an assistant professor in mechanical and industrial engineering and engineering science, who discussed the research on his Substack.
"We aimed for our system to grasp human perceptions of brilliance in chess, distinguishing it from mere winning moves."
Current chess AI research mainly focuses on moves that increase the likelihood of winning, which doesn't always make for an exciting game. Skilled human players, however, often make dramatic or imaginative moves, such as sacrificing a piece in a way that initially seems like a mistake but ultimately leads to victory.
The team collaborated with Leela Chess Zero, a leading chess engine that learns through self-play and has played over 1.6 billion games against itself. They also used Maia, a human-like neural network chess engine developed by U of T computer science researchers.
"We used these neural network chess engines to create game trees at various depths," says Kamron Zaidi, a recent U of T Engineering graduate and co-author of the paper. "We extracted numerous features from these game trees and fed them into a neural network trained on the Lichess database of online chess games, which are labeled by human users."
A game tree in chess represents the current state of the board along with all possible moves and counter-moves. Each board position is a node, and the tree expands until the game is won, drawn, or lost.
Starting with small game trees and gradually increasing their size, the researchers found that their neural network could predict whether a move was brilliant with 79% accuracy using test data.
The research, based on Zaidi's undergraduate engineering science thesis supervised by Guerzhoy, was presented at the International Conference on Computational Creativity in Jönköping, Sweden.
"People from around the world presented research on traditional aspects of creativity, but we all focused on how AI can enhance our understanding and interaction with creativity," says Zaidi.
The work has garnered media attention, with English chess grandmaster Matthew Sadler suggesting that a model capable of recognizing brilliance could serve as a training tool for professionals and provide a more entertaining opponent for amateur players.
The team believes their system has broad applications in understanding creativity and brilliance.
"One of my main interests is defining what we perceive as creativity," says Guerzhoy. "Not just in board games, but in other creative fields like music and art, where formal frameworks and rules exist. Highly creative work involves planning and exploring possibilities."
"But everyone I've spoken to since the paper was published wants to know when they can play against our brilliant chess engine. So, making that possible is our next step."
AI
Zaker Adham
09 November 2024
AI
Zaker Adham
09 November 2024
AI
Zaker Adham
07 November 2024
AI
Zaker Adham
06 November 2024